With artificial intelligence (AI) and computer learning hot subjects just now, it seemed like a good time to revisit Final Jeopardy: Man vs. Machine and the Quest to Know Everything by Stephen Baker. This 2011 book is about a machine taking part in a special edition of the Jeopardy TV game show, but what does it tell us about the future of computers and the role they’ll play in our lives from now on?
The Background to the Book
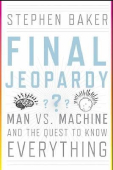
One of the main characters in this story is called Watson, but it isn’t a person. Watson was the name given to a computer system created by IBM that could answer questions asked of it in natural language. While this perhaps doesn’t sound like a big deal now, at the time, it was regarded as a breakthrough in the tech world.
Well, IBM decided to put Watson forward as a contestant on Jeopardy, where it would go up against a couple of champions in, Ken Jennings and Brad Rutter. This face-off was scheduled for a live TV setting, and it’s easy to imagine now that it captured the public’s attention as they waited to see if a computer was really capable of answering questions like a human or even better.
The book was originally released as an e-book just before the match began, with an extra chapter released later to give the result and analyze the outcome. While we now know the result in advance (spoiler alert: the match was won by Watson), it’s still a very interesting read.
The Flow of the Story
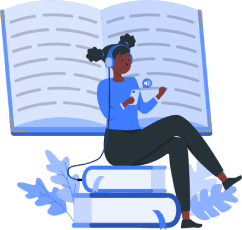
The main pleasure we got from reading this book is the way that the story flows across different times and places. It begins with IBM technicians working away in their offices and labs on new types of smart machines and takes us all the way to the famous Jeopardy studio, where their creation is going to stand or fall based on how well it’s been designed.
Baker does a fine job of bringing all the different characters to life, and, to be fair, they make for an interesting bunch. From the top Jeopardy players to the IBM geniuses behind Watson and the TV executives looking to put on a show, it’s the type of story where real life is arguably better than fiction.
This type of man vs machine contest has always fascinated us. You might recall that IBM’s Deep Blue computer beat chess grandmaster Garry Kasparov in a match that was many people’s introduction to this kind of event. The rapid evolution of AI means that we’re now more used to the idea of people taking on computers in this way, but in 2011, this was a far more exotic idea than it is now.
It probably seemed impossible to many Jeopardy fans that a computer could beat one of their favorites. After all, winning the show requires a vast array of different types of knowledge. It’s not exactly like sitting down to play a chess match where it can have all of the possible moves memorized and analyzed in advance. This delicious sense of breaking new ground and challenging our existing beliefs runs through the book and gives it a lot of its appeal.
How the Story Unfolds
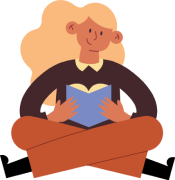
Reading Final Jeopardy: Man vs. Machine and the Quest to Know Everything in 2025 is an interesting concept. It’s fair to say that you won’t approach it like someone reading it the year it was released. We’ve not got the advantage – or disadvantage – of knowing what happens next. There’s no real shock value when the machine wins, in the way that someone in 2011 or 2012 might have been genuinely surprised at the outcome.
It’s to the author’s credit that this doesn’t take anything away from the reading experience. It’s not about the tension of not knowing who wins at this point. Instead, the fun of this book comes from reading about the story as it unfolds and seeing how all the characters interact with one another.
This is an enjoyable read. Before picking up the book, we were worried that it might feel outdated and no longer relevant to a modern audience. It’s not that it’s a particularly old book, but the fast pace of changes in the tech world means that you might approach it with a certain amount of fear over whether it’s now outdated.
The good news is that Baker has packed in enough details and interesting characters to make this a great read from start to finish. You don’t need to be a tech fan or a regular Jeopardy viewer to find plenty of excitement in this story and the way it’s told.
What Does the Book Tell Us About the Future
The final point to cover in this review is around what, if anything, the book tells us about the future. For a start, it lets us see that progress is inevitable. The fact that the machine that’s learned to answer questions like a human take part in a classic, traditional game show like Jeopardy only adds to the feeling that the world is changing faster than most people think.
This book doesn’t focus as mucn on the tech behind Watson as we’d initially hoped for, but that’s fine. This is a story about a smart robot, but it’s not the circuit boards and wires we need to know about; other books cover these areas effectively. This book is about grasping a moment in time when a lot of people realized for the first time that the world was changing in ways they never expected.
Perhaps the lesson to take away from it is that there were lots of people who genuinely believed that a machine could never beat a human in jeopardy or that a robot could drive a car or create art. This story tells us that we shouldn’t believe that someone impossible in the past is going to stay that way forever.